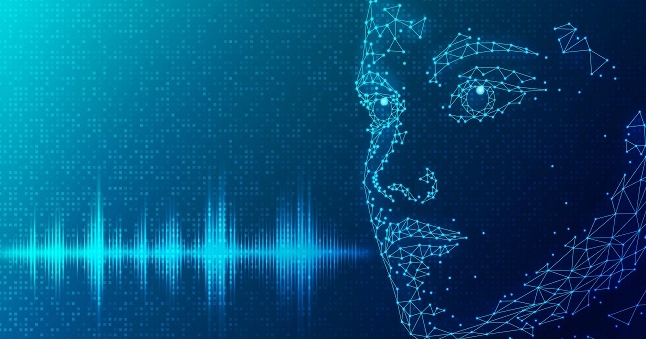
As per the current state of cybersecurity, the focus on deepfakes and large language model (LLM)-powered phishing may be diverting attention from more significant risks related to generative artificial intelligence (GenAI). The concerns around GenAI are less about potential threats from the technology and more about the threats to GenAI from attackers who exploit design weaknesses and flaws in these systems.
Prompt injection, a method used to input text prompts into LLM systems to trigger unintended or unauthorized actions, is identified as a critical adversarial AI threat vector. Venture capital firm SignalFire has identified prompt injection as the number one concern that needs to be addressed urgently in the security marketplace.
Prompt injection is a malicious variation of prompt engineering, where text inputs are crafted to optimize the output of a GenAI system. However, in the case of prompt injection, the intended output usually involves the extraction of sensitive information or triggering undesirable actions. Attackers often persistently prompt the system with follow-up inputs until they can manipulate the LLM to fulfill their objectives, a tactic referred to as social engineering the AI machine.
A comprehensive guide on adversarial AI attacks published by the National Institute of Standards and Technology (NIST) highlighted prompt injection as a major threat, encompassing direct and indirect prompt injection attacks. These attacks can inject malicious input directly into the LLM system’s prompt or manipulate information sources used by the LLM to influence its output.
Moreover, attackers can exploit multimodal GenAI systems that can be prompted by images, extending the scope of prompt injection attacks. This creates challenges in distinguishing between legitimate instructions and user-injected prompts, which can even be in the form of images.
The potential attack vectors using prompt injection are diverse and evolving. Attackers can exploit prompt injection to extract information about how the LLM was trained, override controls preventing the display of inappropriate content, or exfiltrate data from the system or connected sources. This vulnerability in LLMs poses a significant risk to data privacy, potentially exposing sensitive information learned by the system.
Prompt injection attacks can not only compromise sensitive data but also function as a gateway for attackers to manipulate AI systems, triggering undesirable actions embedded in critical systems or processes that use LLMs. As these attacks can essentially unlock a backdoor into the AI’s functionality, the severity of prompt injection danger becomes apparent.
To combat prompt injection, cybersecurity teams need to address the inherent susceptibility of LLMs to input manipulation, which makes it difficult to differentiate between legitimate and malicious prompts. Early attempts in filtering input and setting guardrails on LLMs’ output are in progress. However, these approaches are still in their infancy and susceptible to manipulation, posing a significant challenge to find a foolproof solution to prompt injection attacks.
As the cybersecurity landscape continues to evolve, it is crucial for defenders to address the prompt injection threat promptly and innovate to develop robust solutions. The urgent need to mitigate prompt injection attacks highlights the importance of proactive measures to safeguard LLMs against adversarial AI threats. As the scale of the threat becomes more apparent, the cybersecurity community must work swiftly to stay ahead of attackers leveraging prompt injection.